AI now guides quite a few life-changing selections, from assessing mortgage purposes to determining prison sentences.
Proponents of the strategy argue that it will possibly remove human prejudices, however critics warn that algorithms can amplify our biases — with out even revealing how they reached the choice.
This can lead to AI programs resulting in Black folks being wrongfully arrested, or baby companies unfairly concentrating on poor households. The victims are incessantly from teams which can be already marginalized.
Alejandro Saucedo, Chief Scientist at The Institute for Ethical AI and Engineering Director at ML startup Seldon, warns organizations to consider carefully earlier than deploying algorithms. He instructed TNW his tips about mitigating the dangers.
Explainability
Machine studying programs want to offer transparency. This could be a problem when utilizing highly effective AI fashions, whose inputs, operations, and outcomes aren’t apparent to people.
Explainability has been touted as an answer for years, however efficient approaches stay elusive.
“The machine studying explainability instruments can themselves be biased,” says Saucedo. “For those who’re not utilizing the related instrument or in case you’re utilizing a selected instrument in a approach that’s incorrect or not-fit for goal, you might be getting incorrect explanations. It’s the standard software program paradigm of rubbish in, rubbish out.”
Whereas there’s no silver bullet, human oversight and monitoring can scale back the dangers.
Saucedo recommends figuring out the processes and touchpoints that require a human-in-the-loop. This includes interrogating the underlying knowledge, the mannequin that’s used, and any biases that emerge throughout deployment.
The goal is to determine the touchpoints that require human oversight at every stage of the machine studying lifecycle.
Ideally, this may be certain that the chosen system is fit-for-purpose and related to the use case.
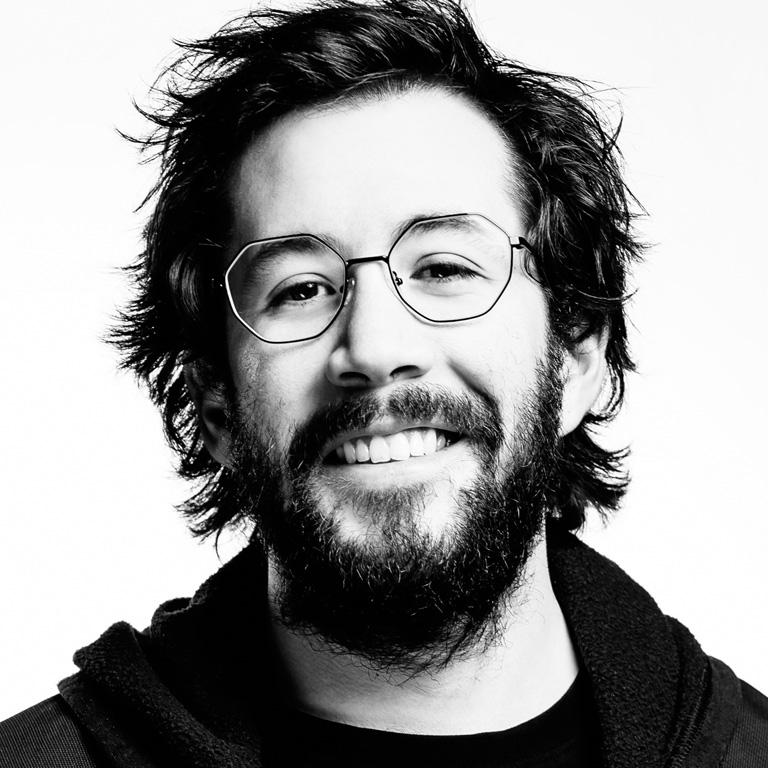
Area specialists may use machine studying explainers to evaluate the prediction of the mannequin, however it’s crucial that they first consider the appropriateness of the system.
“After I say area specialists, I don’t at all times imply technical knowledge scientists,” says Saucedo. “They are often business specialists, coverage specialists, or different people with experience within the problem that’s being tackled.”
Accountability
The extent of human intervention needs to be proportionate to the dangers. An algorithm that recommends songs, as an illustration, gained’t require as a lot oversight as one which dictates bail circumstances.
In lots of instances, a complicated system will solely enhance the dangers. Deep studying fashions, for instance, can add a layer of complexity that causes extra issues than it solves.
“For those who can’t perceive the ambiguities of a instrument you’re introducing, however you do perceive that the dangers have excessive stakes, that’s telling you that it’s a danger that shouldn’t be taken,” says Saucedo.
The operators of AI programs should additionally justify the organizational course of across the fashions they introduce.
This requires an evaluation of the whole chain of occasions that results in a call, from procuring knowledge to the ultimate output.
You want a framework of accountability
“There’s a want to make sure accountability at every step,” says Saucedo. “It’s necessary to be sure that there are greatest practices on not simply the explainability stage, but additionally on what occurs when one thing goes flawed.”
This contains offering a method to investigate the pathway to the end result, knowledge on which area specialists have been concerned, and knowledge on the sign-off course of.
“You want a framework of accountability by strong infrastructure and a sturdy course of that includes area specialists related to the chance concerned at each stage of the lifecycle.”
Safety
When AI programs go flawed, the corporate that deployed them may undergo the implications.
This may be notably damaging when utilizing delicate knowledge, which unhealthy actors can steal or manipulate.
“If artifacts are exploited they are often injected with malicious code,” says Saucedo. “That signifies that when they’re working in manufacturing, they will extract secrets and techniques or share setting variables.”
The software program provide chain provides additional risks.
Organizations that use widespread knowledge science instruments equivalent to TensorFlow and PyTorch introduce further dependencies, which might heighten the dangers.
An improve may trigger a machine studying system to interrupt, and attackers can inject malware on the provide chain stage.
The implications can exacerbate present biases and trigger catastrophic failures.
Saucedo once more recommends making use of greatest practices and human intervention to mitigate the dangers.
An AI system could promise higher outcomes than people, however with out their oversight, the outcomes might be disastrous.
Do you know Alejandro Saucedo, Engineering Director at Seldon and Chief Scientist on the Institute for Moral AI & Machine Studying, is talking on the TNW Convention on June 16? Take a look at the complete record of audio system right here.