Analysis within the subject of machine studying and AI, now a key know-how in virtually each trade and firm, is much too voluminous for anybody to learn all of it. This column, Perceptron (beforehand Deep Science), goals to gather among the most related latest discoveries and papers — significantly in, however not restricted to, synthetic intelligence — and clarify why they matter.
This week in AI, a brand new examine reveals how bias, a standard downside in AI methods, can begin with the directions given to the folks recruited to annotate knowledge from which AI methods study to make predictions. The coauthors discover that annotators choose up on patterns within the directions, which situation them to contribute annotations that then change into over-represented within the knowledge, biasing the AI system towards these annotations.
Many AI methods at this time “study” to make sense of photos, movies, textual content, and audio from examples which were labeled by annotators. The labels allow the methods to extrapolate the relationships between the examples (e.g., the hyperlink between the caption “kitchen sink” and a photograph of a kitchen sink) to knowledge the methods haven’t seen earlier than (e.g., images of kitchen sinks that weren’t included within the knowledge used to “train” the mannequin).
This works remarkably properly. However annotation is an imperfect strategy — annotators convey biases to the desk that may bleed into the educated system. For instance, research have proven that the average annotator is extra prone to label phrases in African-American Vernacular English (AAVE), the casual grammar utilized by some Black Individuals, as poisonous, main AI toxicity detectors educated on the labels to see AAVE as disproportionately poisonous.
Because it seems, annotators’ predispositions may not be solely responsible for the presence of bias in coaching labels. In a preprint study out of Arizona State College and the Allen Institute for AI, researchers investigated whether or not a supply of bias may lie within the directions written by knowledge set creators to function guides for annotators. Such directions sometimes embrace a brief description of the duty (e.g. “Label all birds in these images”) together with a number of examples.
Picture Credit: Parmar et al.
The researchers checked out 14 completely different “benchmark” knowledge units used to measure the efficiency of pure language processing methods, or AI methods that may classify, summarize, translate, and in any other case analyze or manipulate textual content. In learning the duty directions offered to annotators that labored on the information units, they discovered proof that the directions influenced the annotators to comply with particular patterns, which then propagated to the information units. For instance, over half of the annotations in Quoref, a knowledge set designed to check the flexibility of AI methods to grasp when two or extra expressions check with the identical particular person (or factor), begin with the phrase “What’s the title,” a phrase current in a 3rd of the directions for the information set.
The phenomenon, which the researchers name “instruction bias,” is especially troubling as a result of it means that methods educated on biased instruction/annotation knowledge may not carry out in addition to initially thought. Certainly, the coauthors discovered that instruction bias overestimates the efficiency of methods and that these methods usually fail to generalize past instruction patterns.
The silver lining is that giant methods, like OpenAI’s GPT-3, have been discovered to be usually much less delicate to instruction bias. However the analysis serves as a reminder that AI methods, like folks, are inclined to creating biases from sources that aren’t all the time apparent. The intractable problem is discovering these sources and mitigating the downstream affect.
In a much less sobering paper, scientists hailing from Switzerland concluded that facial recognition methods aren’t simply fooled by lifelike AI-edited faces. “Morphing assaults,” as they’re known as, contain the usage of AI to switch the picture on an ID, passport, or different type of id doc for the needs of bypassing safety methods. The coauthors created “morphs” utilizing AI (Nvidia’s StyleGAN 2) and examined them in opposition to 4 state-of-the artwork facial recognition methods. The morphs didn’t submit a big risk, they claimed, regardless of their true-to-life look.
Elsewhere within the laptop imaginative and prescient area, researchers at Meta developed an AI “assistant” that may bear in mind the traits of a room, together with the situation and context of objects, to reply questions. Detailed in a preprint paper, the work is probably going part of Meta’s Project Nazare initiative to develop augmented actuality glasses that leverage AI to research their environment.
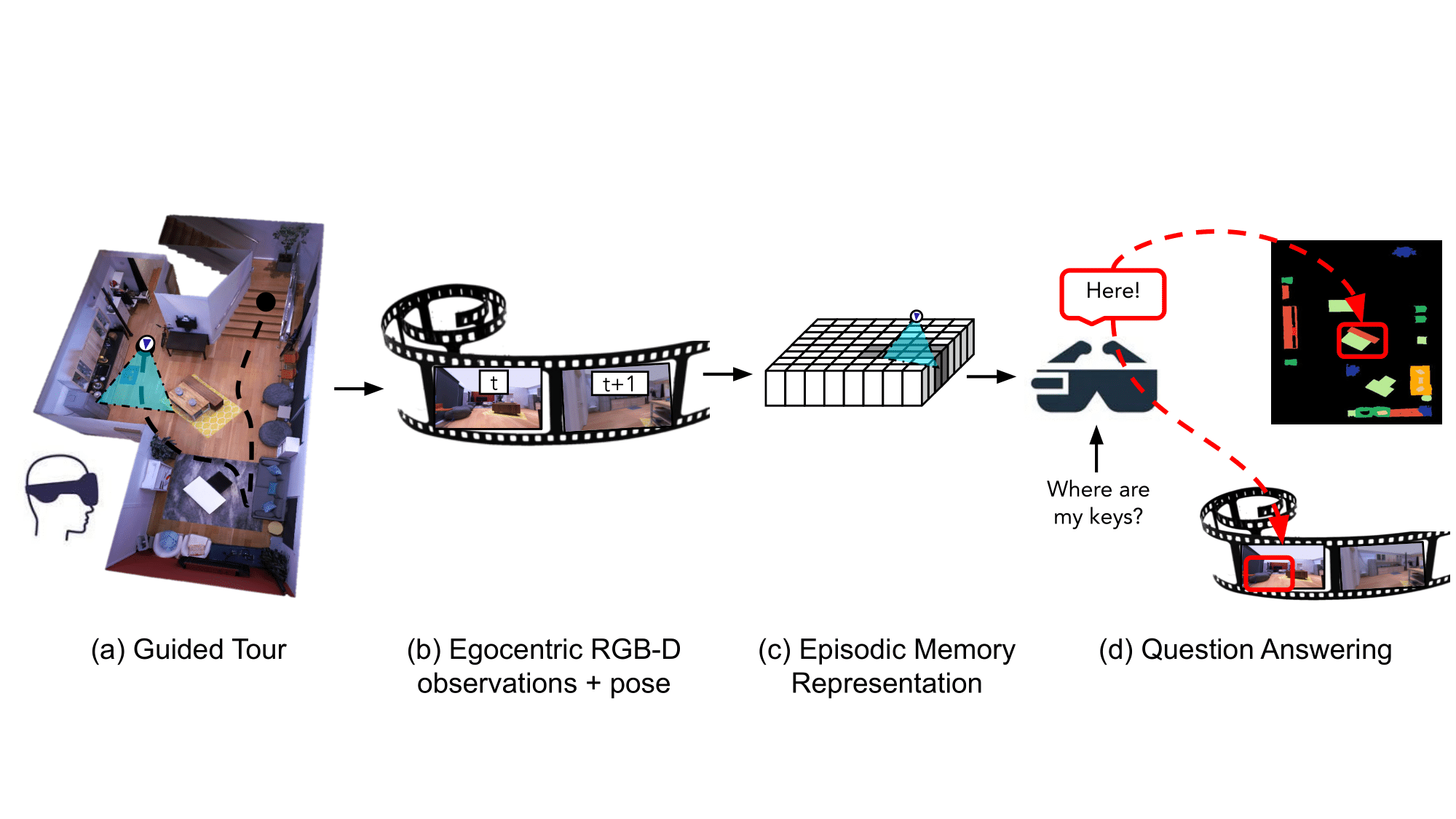
Picture Credit: Meta
The researchers’ system, which is designed for use on any body-worn machine geared up with a digicam, analyzes footage to assemble “semantically wealthy and environment friendly scene reminiscences” that “encode spatio-temporal details about objects.” The system remembers the place objects are and when the appeared within the video footage, and furthermore grounds solutions to questions a consumer may ask in regards to the objects into its reminiscence. For instance, when requested “The place did you final see my keys?,” the system can point out that the keys have been on a aspect desk in the lounge that morning.
Meta, which reportedly plans to launch fully-featured AR glasses in 2024, telegraphed its plans for “selfish” AI final October with the launch of Ego4D, a long-term “selfish notion” AI analysis undertaking. The corporate mentioned on the time that the objective was to show AI methods to — amongst different duties — perceive social cues, how an AR machine wearer’s actions may have an effect on their environment, and the way fingers work together with objects.
From language and augmented actuality to bodily phenomena: an AI mannequin has been helpful in an MIT examine of waves — how they break and when. Whereas it appears a bit of arcane, the reality is wave fashions are wanted each for constructing constructions in and close to the water, and for modeling how the ocean interacts with the ambiance in local weather fashions.
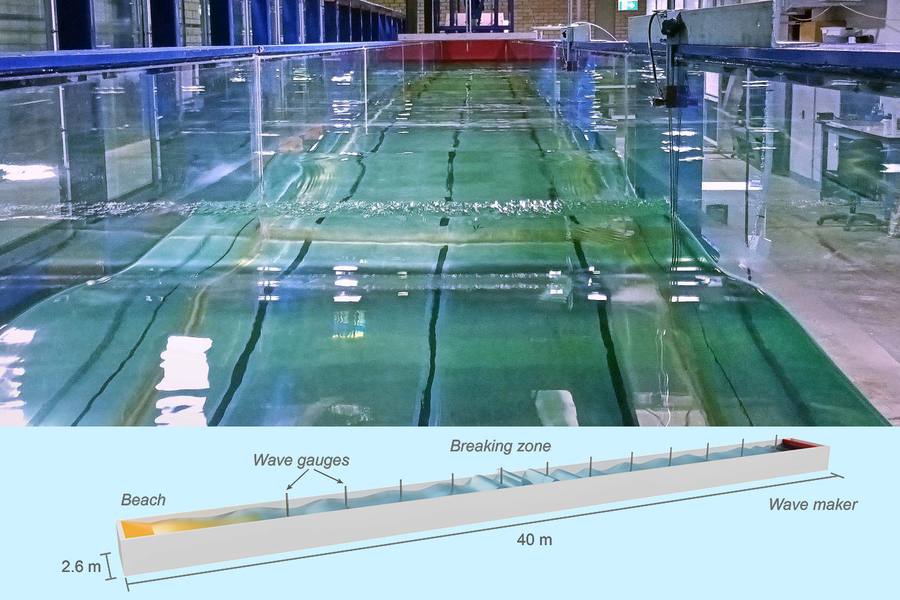
Picture Credit: MIT
Usually waves are roughly simulated by a set of equations, however the researchers trained a machine learning model on a whole lot of wave cases in a 40-foot tank of water stuffed with sensors. By observing the waves and making predictions based mostly on empirical proof, then evaluating that to the theoretical fashions, the AI aided in exhibiting the place the fashions fell brief.
A startup is being born out of analysis at EPFL, the place Thibault Asselborn’s PhD thesis on handwriting evaluation has turned into a full-blown educational app. Utilizing algorithms he designed, the app (known as College Rebound) can establish habits and corrective measures with simply 30 seconds of a child writing on an iPad with a stylus. These are offered to the child within the type of video games that assist them write extra clearly by reinforcing good habits.
“Our scientific mannequin and rigor are necessary, and are what set us other than different present functions,” mentioned Asselborn in a information launch. “We’ve gotten letters from lecturers who’ve seen their college students enhance leaps and bounds. Some college students even come earlier than class to observe.”

Picture Credit: Duke College
One other new discovering in elementary faculties has to do with figuring out listening to issues throughout routine screenings. These screenings, which some readers could bear in mind, usually use a tool known as a tympanometer, which should be operated by educated audiologists. If one isn’t obtainable, say in an remoted faculty district, youngsters with listening to issues could by no means get the assistance they want in time.
Samantha Robler and Susan Emmett at Duke determined to construct a tympanometer that essentially operates itself, sending knowledge to a smartphone app the place it’s interpreted by an AI mannequin. Something worrying can be flagged and the kid can obtain additional screening. It’s not a alternative for an professional, but it surely’s rather a lot higher than nothing and should assist establish listening to issues a lot earlier in locations with out the correct sources.