Analysis within the subject of machine studying and AI, now a key expertise in virtually each trade and firm, is way too voluminous for anybody to learn all of it. This column, Perceptron (beforehand Deep Science), goals to gather among the most related current discoveries and papers — significantly in, however not restricted to, synthetic intelligence — and clarify why they matter.
This week in AI, researchers discovered a way that might permit adversaries to trace the actions of remotely-controlled robots even when the robots’ communications are encrypted end-to-end. The coauthors, who hail from the College of Strathclyde in Glasgow, stated that their examine exhibits adopting one of the best cybersecurity practices isn’t sufficient to cease assaults on autonomous methods.
Distant management, or teleoperation, guarantees to allow operators to information one or a number of robots from afar in a spread of environments. Startups together with Pollen Robotics, Beam, and Tortoise have demonstrated the usefulness of teleoperated robots in grocery shops, hospitals, and places of work. Different corporations develop remotely-controlled robots for duties like bomb disposal or surveying websites with heavy radiation.
However the brand new analysis exhibits that teleoperation, even when supposedly “safe,” is dangerous in its susceptibility to surveillance. The Strathclyde coauthors describe in a paper utilizing a neural community to deduce details about what operations a remotely-controlled robotic is finishing up. After gathering samples of TLS-protected visitors between the robotic and controller and conducting an evaluation, they discovered that the neural community may determine actions about 60% of the time and in addition reconstruct “warehousing workflows” (e.g., choosing up packages) with “excessive accuracy.”
Picture Credit: Shah et al.
Alarming in a much less rapid approach is a brand new study from researchers at Google and the College of Michigan that explored peoples’ relationships with AI-powered methods in international locations with weak laws and “nationwide optimism” for AI. The work surveyed India-based, “financially pressured” customers of immediate mortgage platforms that focus on debtors with credit score decided by risk-modeling AI. In response to the coauthors, the customers skilled emotions of indebtedness for the “boon” of immediate loans and an obligation to just accept harsh phrases, overshare delicate information, and pay excessive charges.
The researchers argue that the findings illustrate the necessity for higher “algorithmic accountability,” significantly the place it issues AI in monetary providers. “We argue that accountability is formed by platform-user energy relations, and urge warning to policymakers in adopting a purely technical method to fostering algorithmic accountability,” they wrote. “As an alternative, we name for located interventions that improve company of customers, allow significant transparency, reconfigure designer-user relations, and immediate a crucial reflection in practitioners in the direction of wider accountability.”
In much less dour research, a crew of scientists at TU Dortmund College, Rhine-Waal College, and LIACS Universiteit Leiden within the Netherlands developed an algorithm that they declare can “resolve” the sport Rocket League. Motivated to discover a much less computationally-intensive approach to create game-playing AI, the crew leveraged what they name a “sim-to-sim” switch method, which skilled the AI system to carry out in-game duties like goalkeeping and putting inside a stripped-down, simplified model of Rocket League. (Rocket League principally resembles indoor soccer, besides with vehicles as an alternative of human gamers in groups of three.)
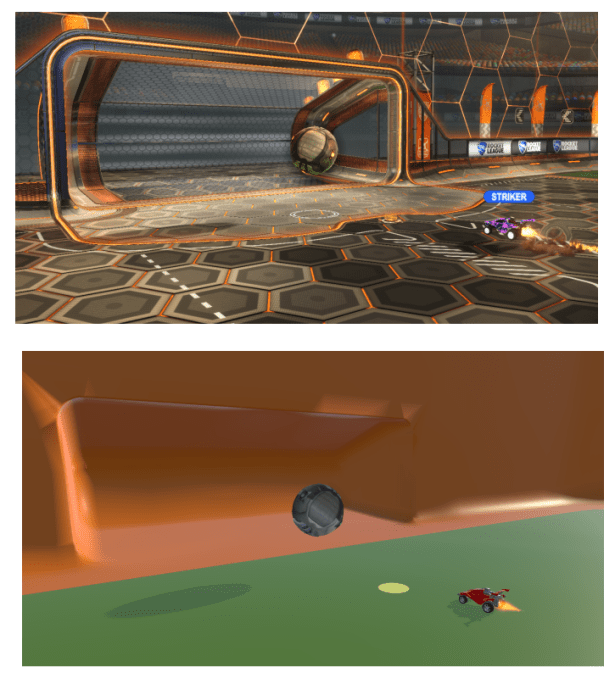
Picture Credit: Pleines et al.
It wasn’t good, however the researchers’ Rocket League-playing system, managed to save lots of almost all photographs fired its approach when goalkeeping. When on the offensive, the system efficiently scored 75% of photographs — a decent file.
Simulators for human actions are additionally advancing at tempo. Meta’s work on monitoring and simulating human limbs has apparent purposes in its AR and VR merchandise, nevertheless it is also used extra broadly in robotics and embodied AI. Analysis that got here out this week bought a tip of the cap from none other than Mark Zuckerberg.
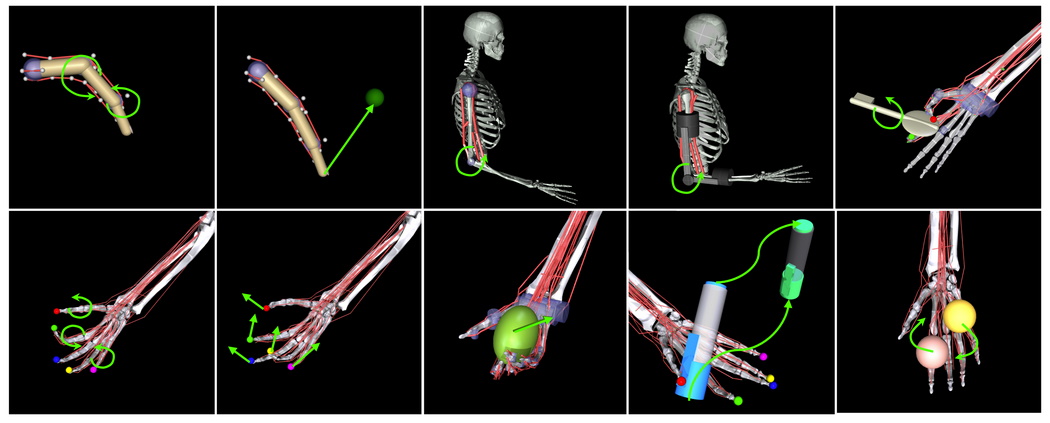
Simulated skeleton and muscle teams in Myosuite.
MyoSuite simulates muscle mass and skeletons in 3D as they work together with objects and themselves — that is necessary for brokers to discover ways to correctly maintain and manipulate issues with out crushing or dropping them, and in addition in a digital world offers real looking grips and interactions. It supposedly runs hundreds of instances sooner on sure duties, which lets simulated studying processes occur a lot faster. “We’re going to open supply these fashions so researchers can use them to advance the sphere additional,” Zuck says. And they did!
Plenty of these simulations are agent- or object-based, however this project from MIT appears at simulating an general system of impartial brokers: self-driving vehicles. The concept is that if in case you have a great quantity of vehicles on the highway, you possibly can have them work collectively not simply to keep away from collisions, however to stop idling and pointless stops at lights.
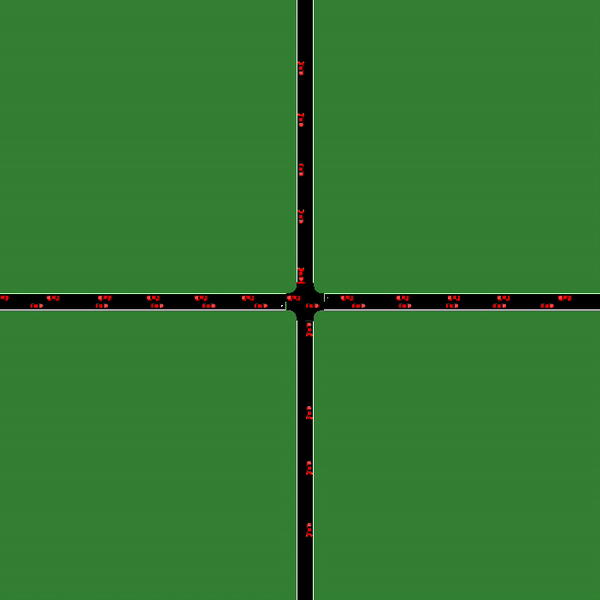
In the event you look intently, solely the entrance vehicles ever actually cease.
As you possibly can see within the animation above, a set of autonomous autos speaking utilizing v2v protocols can principally forestall all however the very entrance vehicles from stopping in any respect by progressively slowing down behind each other, however not a lot that they really come to a halt. This type of hypermiling habits could seem to be it doesn’t save a lot fuel or battery, however once you scale it as much as hundreds or hundreds of thousands of vehicles it does make a distinction — and it may be a extra comfy experience, too. Good luck getting everybody to method the intersection completely spaced like that, although.
Switzerland is taking a great, lengthy have a look at itself — utilizing 3D scanning tech. The nation is making an enormous map utilizing UAVs geared up with lidar and different instruments, however there’s a catch: the motion of the drone (deliberate and unintentional) introduces error into the purpose map that must be manually corrected. Not an issue for those who’re simply scanning a single constructing, however a complete nation?
Fortuitously, a crew out of EPFL is integrating an ML mannequin instantly into the lidar seize stack that may decide when an object has been scanned a number of instances from totally different angles and use that information to line up the purpose map right into a single cohesive mesh. This news article isn’t significantly illuminating, however the paper accompanying it goes into more detail. An instance of the ensuing map is seen within the video above.
Lastly, in surprising however extremely nice AI information, a crew from the College of Zurich has designed an algorithm for tracking animal behavior so zoologists don’t have to clean by way of weeks of footage to seek out the 2 examples of courting dances. It’s a collaboration with the Zurich Zoo, which is sensible when you think about the next: “Our methodology can acknowledge even delicate or uncommon behavioral adjustments in analysis animals, equivalent to indicators of stress, anxiousness or discomfort,” stated lab head Mehmet Fatih Yanik.
So the device may very well be used each for studying and monitoring behaviors in captivity, for the well-being of captive animals in zoos, and for different types of animal research as nicely. They might use fewer topic animals and get extra data in a shorter time, with much less work by grad college students poring over video information late into the evening. Seems like a win-win-win-win state of affairs to me.
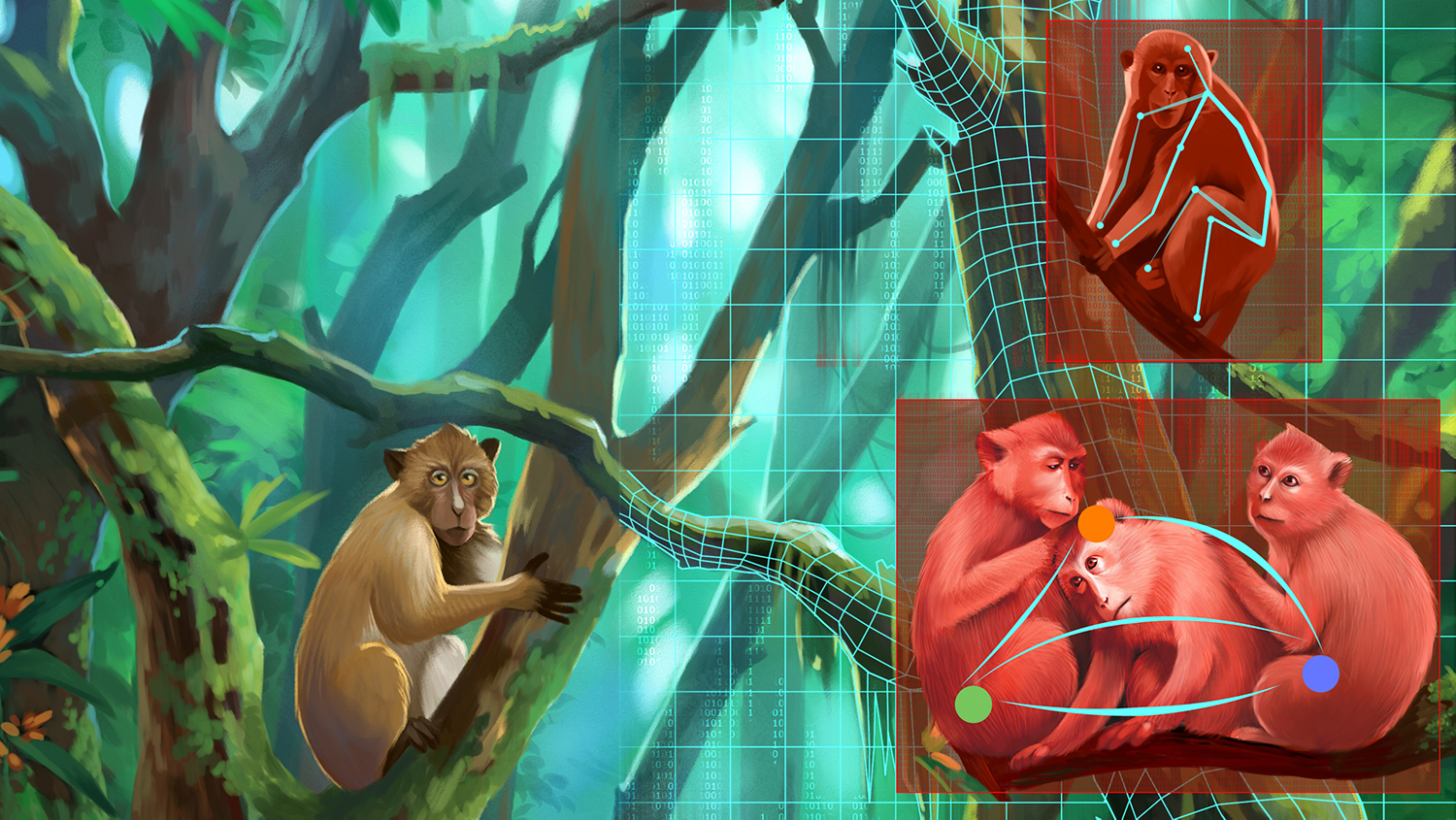
Picture Credit: Ella Marushenko / ETH Zurich
Additionally, love the illustration.