Analysis within the subject of machine studying and AI, now a key know-how in virtually each business and firm, is way too voluminous for anybody to learn all of it. This column, Perceptron (beforehand Deep Science), goals to gather a few of the most related latest discoveries and papers — significantly in, however not restricted to, synthetic intelligence — and clarify why they matter.
This week in AI, a crew of engineers on the College of Glasgow developed “synthetic pores and skin” that may study to expertise and react to simulated ache. Elsewhere, researchers at DeepMind developed a machine studying system that predicts the place soccer gamers will run on a subject, whereas teams from The Chinese language College of Hong Kong (CUHK) and Tsinghua College created algorithms that may generate practical pictures — and even movies — of human fashions.
Based on a press launch, the Glasgow crew’s artificial skin leveraged a brand new sort of processing system primarily based on “synaptic transistors” designed to imitate the mind’s neural pathways. The transistors, constituted of zinc-oxide nanowires printed onto the floor of a versatile plastic, linked to a pores and skin sensor that registered modifications in electrical resistance.
Picture Credit: College of Glasgow
Whereas synthetic pores and skin has been tried earlier than, the crew claims that their design differed in that it used a circuit constructed into the system to behave as an “synthetic synapse” — lowering enter to a spike in voltage. This sped up processing and allowed the crew to “train” the pores and skin how to reply to simulated ache by setting a threshold of enter voltage whose frequency various in keeping with the extent of strain utilized to the pores and skin.
The crew sees the pores and skin being utilized in robotics, the place it might, for instance, forestall a robotic arm from coming into contact with dangerously excessive temperatures.
Tangentially associated to robotics, DeepMind claims to have developed an AI mannequin, Graph Imputer, that may anticipate the place soccer gamers will transfer utilizing digital camera recordings of solely a subset of gamers. Extra impressively, the system could make predictions about gamers past the view of the digital camera, permitting it to trace the place of most — if not all — gamers on the sector pretty precisely.
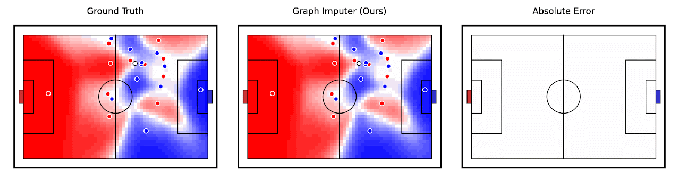
Picture Credit: DeepMind
Graph Imputer isn’t good. However the DeepMind researchers say it could possibly be used for functions like modeling pitch management, or the chance {that a} participant might management the ball assuming it’s at a given location. (A number of main Premier League groups use pitch management fashions throughout video games, in addition to in pre-match and post-match evaluation.) Past soccer and different sports activities analytics, DeepMind expects the strategies behind Graph Imputer can be relevant to domains like pedestrian modeling on roads and crowd modeling in stadiums.
Whereas synthetic pores and skin and movement-predicting methods are spectacular, to make sure, photo- and video-generating methods are progressing at a quick clip. Clearly, there’s high-profile works like OpenAI’s Dall-E 2 and Google’s Imagen. However check out Text2Human, developed by CUHK’s Multimedia Lab, which may translate a caption like “the woman wears a short-sleeve T-shirt with pure coloration sample, and a brief and denim skirt” into an image of an individual who doesn’t really exist.
In partnership with the Beijing Academy of Synthetic Intelligence, Tsinghua College created an much more bold mannequin referred to as CogVideo that may generate video clips from textual content (e.g., “a person in snowboarding,” “a lion is consuming water”). The clips are rife with artifacts and different visible weirdness, however contemplating they’re of fully fictional scenes, it’s onerous to criticize too harshly.
Machine studying is usually utilized in drug discovery, the place the near-infinite number of molecules that seem in literature and principle should be sorted by and characterised to be able to discover probably useful results. However the quantity of knowledge is so massive, and the price of false positives probably so excessive (it’s expensive and time-consuming to chase leads) that even 99% accuracy isn’t ok. That’s particularly the case with unlabeled molecular information, by far the majority of what’s on the market (in contrast with molecules which were manually studied over time).
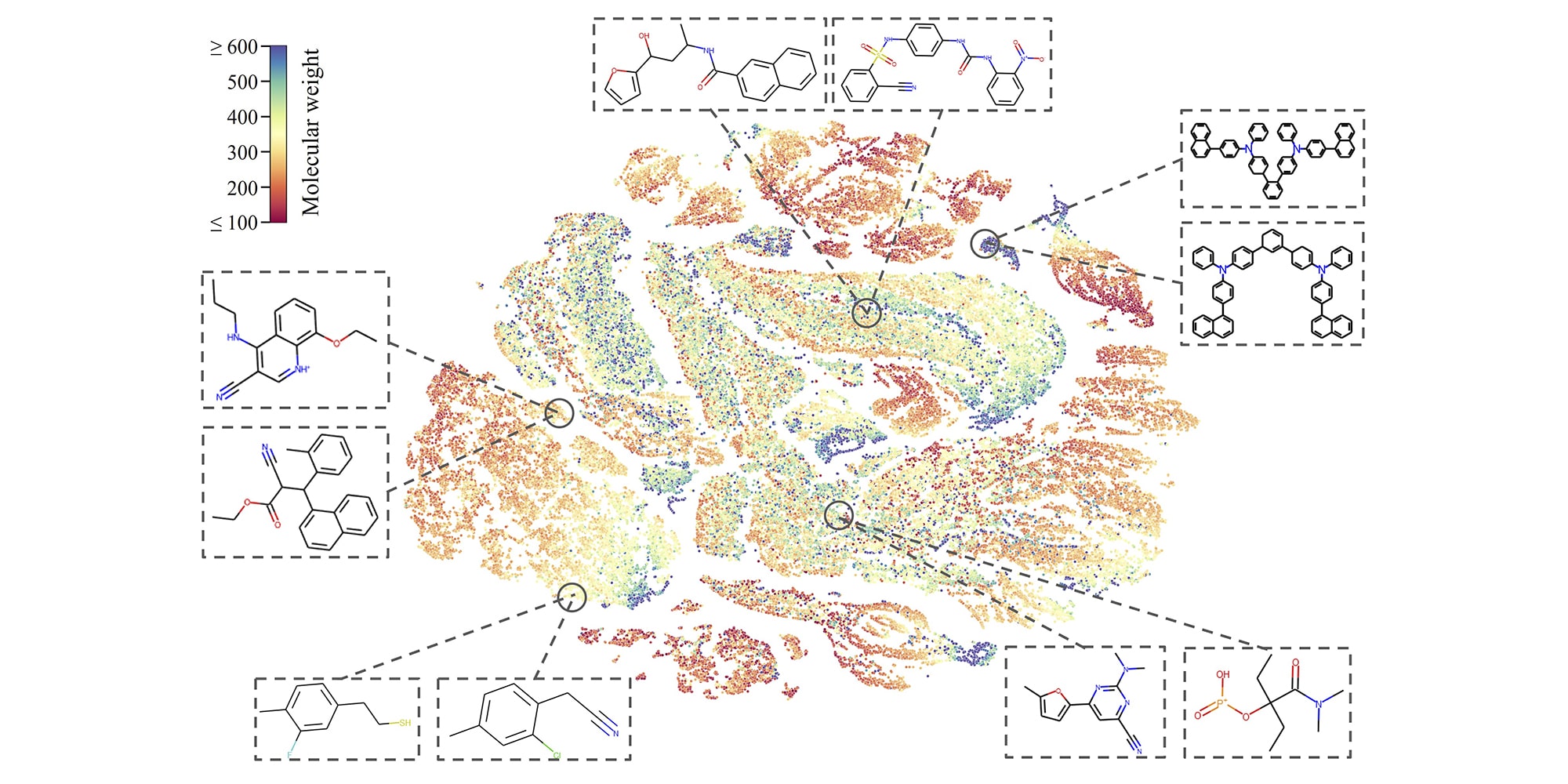
Picture Credit: CMU
CMU researchers have been working to create a mannequin to kind by billions of uncharacterized molecules by coaching it to make sense of them with none further data. It does this by making slight modifications to the (digital) molecule’s construction, like hiding an atom or eradicating a bond, and observing how the ensuing molecule modifications. This lets its study intrinsic properties of how such molecules are fashioned and behave — and led to it outperforming different AI fashions in figuring out poisonous chemical substances in a take a look at database.
Molecular signatures are additionally key in diagnosing illness — two sufferers might current related signs, however cautious evaluation of their lab outcomes exhibits that they’ve very completely different situations. In fact that’s commonplace doctoring apply, however as information from a number of checks and analyses piles up, it will get tough to trace all of the correlations. The Technical College of Munich is engaged on a sort of clinical meta-algorithm that integrates a number of information sources (together with different algorithms) to distinguish between sure liver illnesses with related displays. Whereas such fashions received’t change docs, they’ll proceed to assist wrangle the rising volumes of knowledge that even specialists might not have the time or experience to interpret.