The primary severe accident involving a self-driving automotive in Australia occurred in March this 12 months. A pedestrian suffered life-threatening accidents when hit by a Tesla Model 3, which the driving force claims was in “autopilot” mode.
Within the US, the freeway security regulator is investigating a series of accidents the place Teslas on autopilot crashed into first-responder autos with flashing lights throughout visitors stops.
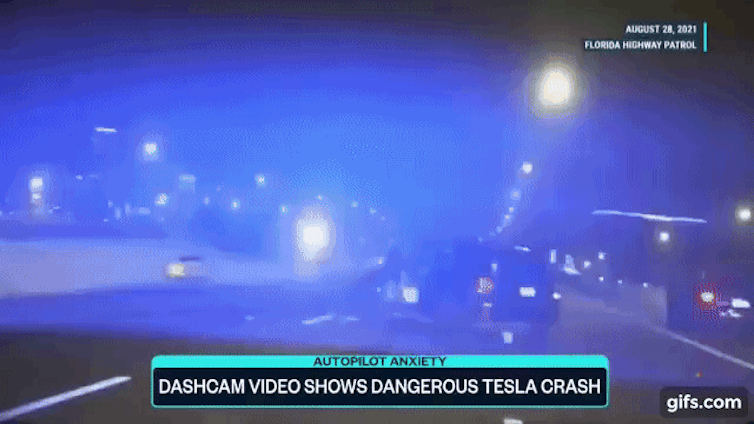
NBC / YouTube
The choice-making processes of “self-driving” automobiles are sometimes opaque and unpredictable (even to their producers), so it may be arduous to find out who ought to be held accountable for incidents resembling these. Nonetheless, the rising discipline of “explainable AI” could assist present some solutions.
Who’s accountable when self-driving automobiles crash?
Whereas self-driving automobiles are new, they’re nonetheless machines made and offered by producers. Once they trigger hurt, we should always ask whether or not the producer (or software program developer) has met their security duties.
Fashionable negligence legislation comes from the well-known case of Donoghue v Stevenson, the place a lady found a decomposing snail in her bottle of ginger beer. The producer was discovered negligent, not as a result of he was anticipated to instantly predict or management the habits of snails, however as a result of his bottling course of was unsafe.
By this logic, producers and builders of AI-based programs like self-driving automobiles could not be capable to foresee and management all the pieces the “autonomous” system does, however they’ll take measures to cut back dangers. If their danger administration, testing, audits, and monitoring practices are usually not ok, they need to be held accountable.
How a lot danger administration is sufficient?
The tough query can be “How a lot care and the way a lot danger administration is sufficient?” In complicated software program, it’s impossible to test for every bug prematurely. How will builders and producers know when to cease?
Luckily, courts, regulators, and technical requirements our bodies have expertise in setting requirements of care and accountability for dangerous however helpful actions.
Requirements might be very exacting, just like the European Union’s draft AI regulation, which requires dangers to be lowered “so far as attainable” with out regard to price. Or they could be extra like Australian negligence legislation, which allows much less stringent administration for much less probably or much less extreme dangers, or the place danger administration would scale back the general advantage of the dangerous exercise.
Authorized instances can be sophisticated by AI opacity
As soon as we’ve a transparent customary for dangers, we want a approach to implement it. One method might be to offer a regulator powers to impose penalties (because the ACCC does in competitors instances, for instance).
People harmed by AI programs should additionally be capable to sue. In instances involving self-driving automobiles, lawsuits towards producers can be notably essential.
Nonetheless, for such lawsuits to be efficient, courts might want to perceive intimately the processes and technical parameters of the AI programs.
Producers usually favor to not reveal such particulars for business causes. However courts have already got procedures to stability business pursuits with an applicable quantity of disclosure to facilitate litigation.
A larger problem could come up when AI programs themselves are opaque “black boxes”. For instance, Tesla’s autopilot performance depends on “deep neural networks”, a well-liked kind of AI system by which even the builders can by no means be solely certain how or why it arrives at a given consequence.
‘Explainable AI’ to the rescue?
Opening the black field of contemporary AI programs is the main focus of a new wave of pc science and humanities scholars: the so-called “explainable AI” motion.
The purpose is to assist builders and end-users perceive how AI programs make selections, both by altering how the programs are constructed or by producing explanations after the very fact.
In a classic example, an AI system mistakenly classifies an image of a husky as a wolf. An “explainable AI” methodology reveals the system centered on snow within the background of the picture, quite than the animal within the foreground.
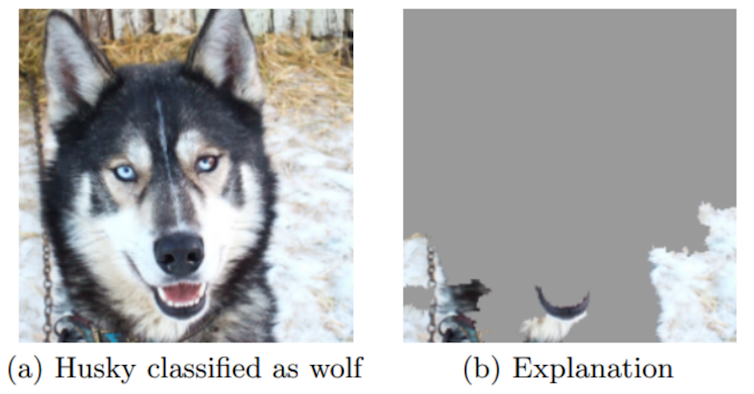
How this is likely to be utilized in a lawsuit will depend upon numerous components, together with the precise AI know-how and the hurt precipitated. A key concern can be how a lot entry the injured social gathering is given to the AI system.
The Trivago case
Our new research analyzing an essential latest Australian courtroom case supplies an encouraging glimpse of what this might seem like.
In April 2022, the Federal Courtroom penalized international lodge reserving firm Trivago $44.7 million for deceptive clients about lodge room charges on its web site and in TV promoting, after a case introduced on by competition watchdog the ACCC. A essential query was how Trivago’s complicated rating algorithm selected the top-ranked provide for lodge rooms.
The Federal Courtroom arrange guidelines for proof discovery with safeguards to guard Trivago’s mental property, and each the ACCC and Trivago referred to as professional witnesses to supply proof explaining how Trivago’s AI system labored.
Even with out full entry to Trivago’s system, the ACCC’s professional witness was in a position to produce compelling proof that the system’s habits was not in step with Trivago’s declare of giving clients the “greatest worth”.
This exhibits how technical specialists and legal professionals collectively can overcome AI opacity in courtroom instances. Nonetheless, the method requires shut collaboration and deep technical experience, and can probably be costly.
Regulators can take steps now to streamline issues sooner or later, resembling requiring AI firms to adequately doc their programs.
The highway forward
Autos with various degrees of automation have gotten extra frequent, and totally autonomous taxis and buses are being examined each in Australia and overseas.
Holding our roads as protected as attainable would require shut collaboration between AI and authorized specialists, and regulators, producers, insurers, and customers will all have roles to play.
This text by Aaron J. Snoswell, Submit-doctoral Analysis Fellow, Computational Legislation & AI Accountability, Queensland University of Technology; Henry Fraser, Analysis Fellow in Legislation, Accountability and Knowledge Science, Queensland University of Technology, and Rhyle Simcock, PhD Candidate, Queensland University of Technology is republished from The Conversation underneath a Artistic Commons license. Learn the original article.